Machine learning is a branch of artificial intelligence which is rapidly transforming numerous sectors, revolutionising the way we interact with technology. At the forefront of this revolution is the media industry, where machine learning is not just an accessory but a driving force in content creation and manipulation. Its capacity to analyse and learn from vast datasets is enabling groundbreaking advancements, particularly in the realm of audiovisual media. Among these innovations, one that stands out is the creation and detection of deepfakes, which epitomises both the incredible potential and the daunting challenges posed by AI in media.
Deepfakes represent a cutting-edge application of AI in the media industry, where video or audio recordings are altered so convincingly that they seem real. The term ‘deepfake’ is a blend of “deep learning” and “fake,” indicating its reliance on deep learning, a subset of machine learning algorithms, for creating fabricated content. Deepfakes are typically generated by using sophisticated artificial intelligence techniques to superimpose existing images and videos onto source images or videos. This involves training an algorithm on a large dataset of facial images to understand how to manipulate facial expressions and movements convincingly.
The realism of deepfakes stems from their ability to replicate nuances in human expressions, making it challenging to distinguish between real and altered content. While initially, creating deepfakes required substantial technical expertise and computing power, advancements in AI have made this technology more accessible. Consequently, deepfakes have found applications ranging from entertainment to malicious uses, including creating realistic-looking fake news and impersonating public figures. The growing prevalence of deepfakes raises significant ethical and legal concerns, highlighting the need for effective detection and regulation strategies to combat the potential misuse of this powerful AI tool.
The technology underpinning deepfakes primarily involves Generative Adversarial Networks (GANs), a remarkable innovation in the field of machine learning. GANs consist of two neural networks, namely the generator and the discriminator, engaged in a continuous competition. The generator creates images or videos, while the discriminator evaluates their authenticity. The process begins with feeding the generator an extensive dataset of real images or videos, enabling it to learn and replicate the nuances of human features and movements.
As the generator produces fake content, the discriminator, trained on real data, evaluates its authenticity. If the discriminator identifies the content as fake, it sends feedback to the generator, which then tweaks its algorithms to improve the realism of its output. This iterative process continues until the generator creates content that the discriminator can no longer easily distinguish from real data. The result is a deepfake that is often indistinguishable from authentic content to the naked eye.
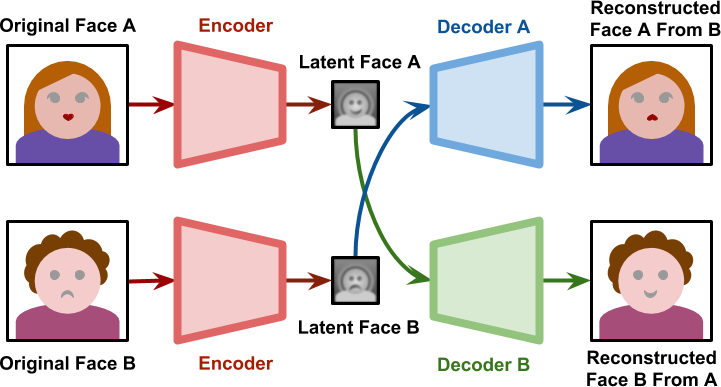
The intricacy of GANs lies in their ability to learn from and adapt to the subtlest aspects of human expressions and environments, such as lighting, shadows, and textures. They can also assimilate and mimic vocal patterns, making it possible to create not only visually convincing deepfakes but also audio deepfakes that can replicate a person’s voice with high accuracy. The advancements in GANs have significantly lowered the barriers to creating deepfakes, making this technology more accessible and consequently, more prevalent.
Deepfakes, despite their potential for misuse, have several positive applications, particularly in the media and entertainment industry. They offer novel ways to enhance film production, such as de-aging actors or resurrecting historical figures for biographical films. This can lead to more immersive and engaging storytelling, allowing filmmakers to depict scenes and characters that would otherwise be impossible or impractical to film. Additionally, deepfakes are being used in creating personalised video greetings and interactive historical documentaries, offering a unique and engaging user experience. These applications demonstrate the vast creative potential of deepfakes, extending beyond mere replication to innovative content creation.
The advent of deepfakes has introduced a formidable tool for creating disinformation and spreading fake news, posing a significant threat to the integrity of information in the digital age. With their ability to fabricate convincing audio and video content, deepfakes can be used to create false narratives, impersonate public figures, and manipulate public opinion. This is particularly concerning in the context of political discourse and elections, where deepfakes can be employed to discredit individuals.
The potential of deepfakes to influence public perception is not limited to politics; it extends to social, cultural, and international relations. Fabricated content can be used to create fictitious events, stir unrest, or exacerbate tensions between groups or nations. The challenge in combating this form of digital deceit lies in the sophistication of the technology, which makes detection difficult for the average person. As deepfakes become more realistic and easier to produce, the risk of their use as a tool for misinformation and propaganda increases, necessitating vigilant and sophisticated measures to identify and mitigate their spread.
Beyond politics and social discourse, deepfakes pose significant economic implications. They have the potential to disrupt financial markets through fabricated statements or announcements from corporate leaders or influential financial figures. A deepfake video or audio clip mimicking a CEO making a false statement about a company’s financial health or business strategy could lead to drastic fluctuations in stock prices or market instability. This not only undermines the trust in public communications from businesses and financial institutions but also opens up avenues for fraudulent activities and market manipulation, highlighting the urgent need for robust verification mechanisms in the financial sector.
As deepfakes become increasingly sophisticated, detecting them poses a significant challenge. The same AI technologies that empower their creation are also essential in their detection. Researchers and tech companies are continually developing machine learning models to identify deepfakes, relying on subtle clues often imperceptible to the human eye. These detection methods analyse various aspects such as facial expressions, eye movements, skin texture, and even breathing patterns for inconsistencies that might indicate manipulation.
One of the key techniques involves examining the lack of coherence in facial expressions or physical movements. AI algorithms can detect unnatural blinking patterns or facial movements that don’t align with normal human expressions. Additionally, inconsistencies in lighting, shadows, and background noise in audio recordings are telltale signs of manipulation that advanced algorithms can identify. Another promising approach is the analysis of deepfake videos’ metadata, which can reveal anomalies in the file’s creation or editing history.
However, the detection of deepfakes is a constantly evolving arms race. As detection methods improve, so do the techniques for creating more convincing deepfakes. This continuous improvement loop underscores the necessity for ongoing research and development in deepfake detection technologies. Collaborative efforts between AI researchers, cybersecurity experts, and policymakers are crucial in developing more effective tools to combat this threat.
Machine learning’s role in the media, especially in the context of deepfakes, is a paradigmatic example of a double-edged sword. While it offers incredible possibilities for creative and innovative content creation, it simultaneously poses serious risks in terms of misinformation, political manipulation, and economic disruption. The ongoing challenge lies in balancing the beneficial applications of this technology while mitigating its potential for harm. This balance necessitates continuous advancements in detection techniques, increased public awareness, and comprehensive legislative measures. Ensuring the responsible use of machine learning in media is imperative for maintaining trust and integrity in the digital information age.